Most of living beings have sensory organs to measure their movements in spaces. Especially vestibular system of vertebrates, that measures translations and rotations. More simple animals, such as insects, have sensitive vibrissa that measure relative wind speed. The fact that most of animals have such a sensory system indicates that this system gives a decisive advantage that leads to its generalization through evolution.
The advantage of such a sensory organ is based on the fact that the theoretical space of possible interactions has more dimensions than the physical space. Indeed, two interactions can produce a same movement in space. We cannot exploit directly the information from the vestibular system: as it can differ from individual to another, it is not possible to suppose that sensori stimuli and movements in spaces are known a priori. However, we can suppose that each interaction produces a unique vestibular stimulus. Indeed, the enaction of an interaction implies that the associated movement was performed. Thus, it is possible to gather interactions that produce a same movement.
We implemented and tested a variant of our space memory that takes information from a mechanism that measure movements of the agent into account. This mechanism gives, for each enacted interaction, the performed movement (without any semantics nor signification a priori).
Composite interactions are modified: as the path correspond to the movement required to reach an object, we can consider a path as a sequence of movements rather than a sequence of interactions. A composite interaction thus consists of a sequence of interactions that characterizes the object that affords this sequence, preceded by a sequence of movements that characterizes the position of this object:
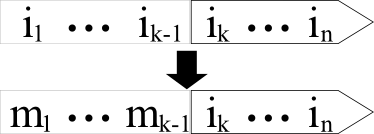
The utilization of movements to characterize the path reduces the number of possible composite interactions, as several sequences of interactions can generate a same sequence of movements. However, as the path does not consist in a sequence of interactions anymore, we cannot define a priori the satisfaction value of a composite interaction. It is not possible to determine if the path is enactable, as more than one sequence can generate the sequence of movements. It is thus needed to define a sequence of interactions that matches the path of this interaction. We adapted the selection mechanism to generates the path of a composite interaction according to the content of the space memory. Generating the path of a composite interaction consists in defining a set of enactable composite interactions included in the path of this interaction such as each movement of the path can be replaced by a primitive interaction.
Note that when more than one interaction can replace a movement, the mechanism selects the interaction with the greatest satisfaction value. The sequence that compose the path is thus the sequence with the greatest satisfaction value. The satisfaction value of a composite interaction is defined according to the reconstituted interaction, and computed as the sum of satisfaction values of primitive interactions that compose it.
A variant of the previous experiment that implements the vestibular system shows a significant improvement of interaction signature learning process. The learning process is faster, and, as composite interactions are less numerous and more often tested, signatures are more coherent with objects that afford interactions.
The experiment is conducted in the same conditions than for the version without vestibular system : we let the agent learns signatures of its composite interactions until the stabilization of its behavior.
We can observe that the learning duration is considerably lowered, even if it depends on the used configuration. In the small loop configuration, for example, with a composite interaction length limitation of 2, the minimum number of cycle for behavior stabilisation is reduced from 4000 to 1800 cycles, and with a length limit of 3, it is reduced from 35 500 to 5800 cycles. It was also possible to test the learning process with a length limit of 4 in a reasonable amount of time: between 40 600 and 50 000 cycles are needed to obtain a stable behavior, which is equivalent to a signature learning of the non vestibular system with a length limit of 3.
The percentage of reliable and correct interactions is also greater. This is due to the fact that composite interactions are more often tested, and to the lower number of possible composite interactions. The possibility to enact a path is less influenced by the context of the agent, as two opposite interactions can generate a same movement.
The specialisation according to positions and elements of the environement is also more precise (Figure 2). The agent clearly identified positions and objects it can observe. We can note that with a length limit of 4, the specialization is greater than with the non-vestibular mechanism using a length limit of 3, even with a greater number of composite interactions. We can also note that most of non-specialized interactions are related to objects that escape the agent's interactional system.
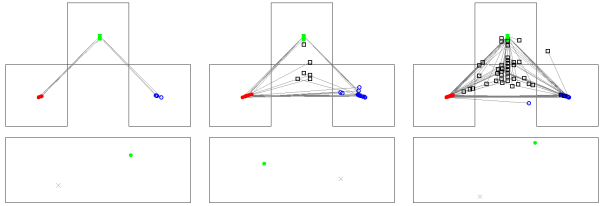
However, we do not observe significant changes in the agent's behavior. The agent uses the same sequences as previously. We can note that with a length limit of 4, the agent still uses the same interactions of length 2. Indeed, most of interactions of length 3 or 4 are afforded by objects that escape the sensory system of the agent, and for which it is not possible to define the signature. We also observe the same errors than previously, but these error are fewer.
This experiment shows that using a vestibular system on an agent increase significantly performances in learning of a space memory, without variation nor drop in performances of the agent's behavior.